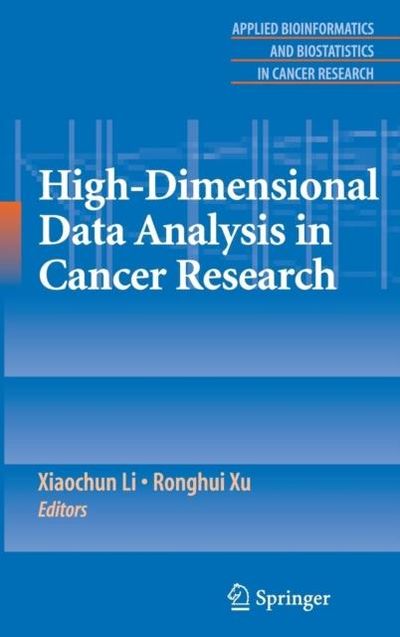
High-Dimensional Data Analysis in Cancer Research Hard cover - 2008
by Xiaochun Li
- New
- Hardcover
Description
Standard delivery: 7 to 12 days
Details
- Title High-Dimensional Data Analysis in Cancer Research
- Author Xiaochun Li
- Binding Hard Cover
- Edition U. S. EDITION
- Condition New
- Pages 392
- Volumes 1
- Language ENG
- Publisher Springer, New York, NY
- Date 2008-12-12
- Illustrated Yes
- Features Bibliography, Illustrated, Index, Table of Contents
- Bookseller's Inventory # ria9780387697635_pod
- ISBN 9780387697635 / 0387697632
- Weight 0.88 lbs (0.40 kg)
- Dimensions 9.3 x 6.1 x 0.6 in (23.62 x 15.49 x 1.52 cm)
- Library of Congress subjects Neoplasms, Multivariate analysis
- Library of Congress Catalog Number 2008940562
- Dewey Decimal Code 616.994
About Ria Christie Collections Greater London, United Kingdom
Hello We are professional online booksellers. We sell mostly new books and textbooks and we do our best to provide a competitive price. We are based in Greater London, UK. We pride ourselves by providing a good customer service throughout, shipping the items quickly and replying to customer queries promptly. Ria Christie Collections
30 day return guarantee, with full refund including original shipping costs for up to 30 days after delivery if an item arrives misdescribed or damaged.
From the publisher
From the rear cover
With the advent of high-throughput technologies, various types of high-dimensional data have been generated in recent years for the understanding of biological processes, especially processes that relate to disease occurrence or management of cancer. Motivated by these important applications in cancer research, there has been a dramatic growth in the development of statistical methodology in the analysis of high-dimensional data, particularly related to regression model selection, estimation and prediction.
High-Dimensional Data Analysis in Cancer Research, edited by Xiaochun Li and Ronghui Xu, is a collective effort to showcase statistical innovations for meeting the challenges and opportunities uniquely presented by the analytical needs of high-dimensional data in cancer research, particularly in genomics and proteomics. All the chapters included in this volume contain interesting case studies to demonstrate the analysis methodology.
High-Dimensional Data Analysis in Cancer Research is an invaluable reference for
researchers, statisticians, bioinformaticians, graduate students and data analysts working in the fields of cancer research.