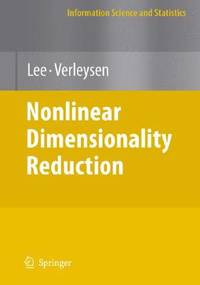
Nonlinear Dimensionality Reduction (Information Science and Statistics) Hardcover - 2007 - 2007th Edition
by Lee, John A
- Used
- Good
- Hardcover
Description
Details
- Title Nonlinear Dimensionality Reduction (Information Science and Statistics)
- Author Lee, John A
- Binding Hardcover
- Edition number 2007th
- Edition 2007
- Condition Used - Good
- Pages 309
- Volumes 1
- Language ENG
- Publisher Springer
- Date 2007-12-05
- Illustrated Yes
- Features Bibliography, Illustrated, Index, Table of Contents
- Bookseller's Inventory # 0387393501.G
- ISBN 9780387393506 / 0387393501
- Weight 1.4 lbs (0.64 kg)
- Dimensions 9.3 x 6.4 x 1 in (23.62 x 16.26 x 2.54 cm)
- Library of Congress subjects Computational complexity, Visualization
- Library of Congress Catalog Number 2006939149
- Dewey Decimal Code 511.352
About Bonita California, United States
From the publisher
From the rear cover
Methods of dimensionality reduction provide a way to understand and visualize the structure of complex data sets. Traditional methods like principal component analysis and classical metric multidimensional scaling suffer from being based on linear models. Until recently, very few methods were able to reduce the data dimensionality in a nonlinear way. However, since the late nineties, many new methods have been developed and nonlinear dimensionality reduction, also called manifold learning, has become a hot topic. New advances that account for this rapid growth are, e.g. the use of graphs to represent the manifold topology, and the use of new metrics like the geodesic distance. In addition, new optimization schemes, based on kernel techniques and spectral decomposition, have lead to spectral embedding, which encompasses many of the recently developed methods.
This book describes existing and advanced methods to reduce the dimensionality of numerical databases. For each method, the description starts from intuitive ideas, develops the necessary mathematical details, and ends by outlining the algorithmic implementation. Methods are compared with each other with the help of different illustrative examples.
The purpose of the book is to summarize clear facts and ideas about well-known methods as well as recent developments in the topic of nonlinear dimensionality reduction. With this goal in mind, methods are all described from a unifying point of view, in order to highlight their respective strengths and shortcomings.
The book is primarily intended for statisticians, computer scientists and data analysts. It is also accessible to other practitioners having a basic background in statistics and/or computational learning, like psychologists (in psychometry) and economists.
John A. Lee is a Postdoctoral Researcher of the Belgian National Fund for Scientific Research (FNRS). He is (co-)author of more than 30 publications in the field ofmachine learning and dimensionality reduction. Michel Verleysen is Professor at the Universit catholique de Louvain (Louvain-la-Neuve, Belgium), and Honorary Research Director of the Belgian National Fund for Scientific Research (FNRS). He is the chairman of the annual European Symposium on Artificial Neural Networks, co-editor of the Neural Processing Letters journal (Springer), and (co-)author of more than 200 scientific publications in the field of machine learning.